- Learn About Me
About Me
As a Data Science and Business Analytics Master’s student, I specialize in turning complex data into actionable insights for business growth. My expertise includes statistical analysis, machine learning, and AI, with a focus on Deep Learning and Generative AI. With a background in data science and finance, I excel in predictive modeling and optimizing business processes.I’ve led projects focused on advanced data science and machine learning applications. I’m now seeking a PFE Internship to apply my skills in AI, machine learning, and business analytics within a dynamic team.
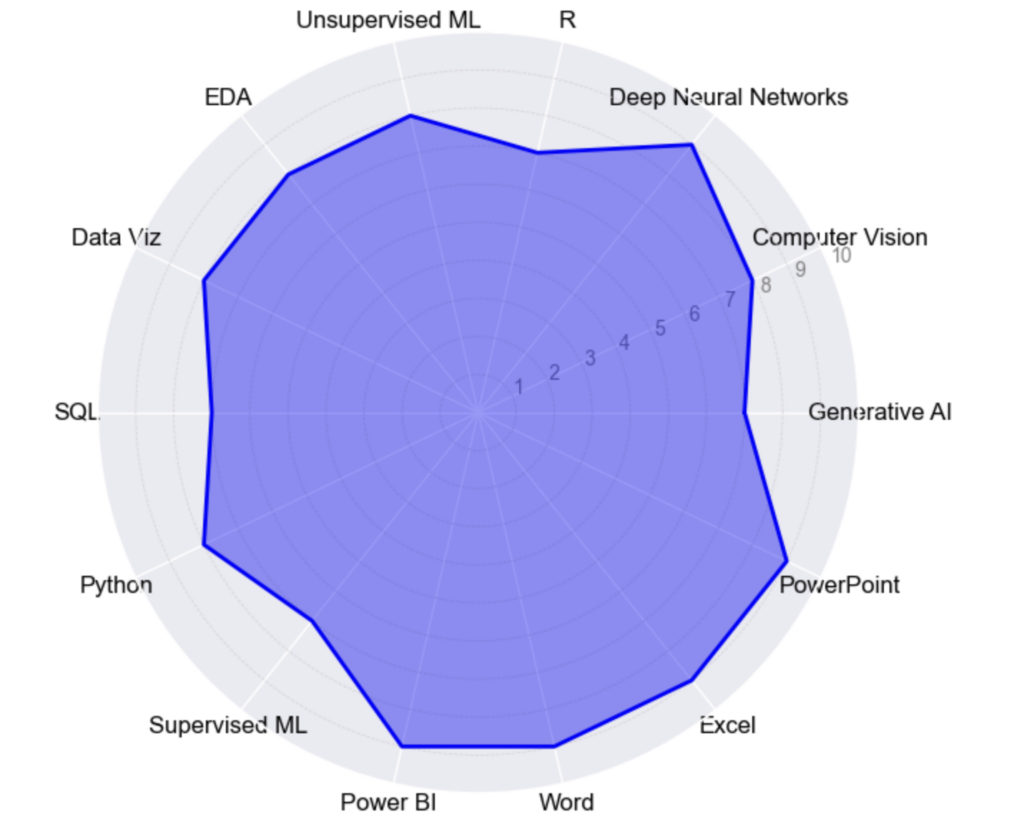
- My journey in knowledge
Education
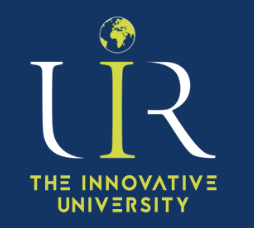
Université Internationale de Rabat, Maroc : 2023 – 2025
Master in Data Science & Business Analytics : GPA: 3.5/4
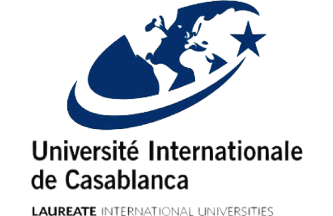
Université Internationale de Casablanca, Maroc : 2020 – 2023
Bachelor in Management – Finance
Distinction: Very Good
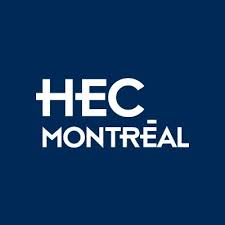
HEC Montréal, Canada : 2018 – 2019
Preparatory Year in Business Administration
CERTIFICATIONS
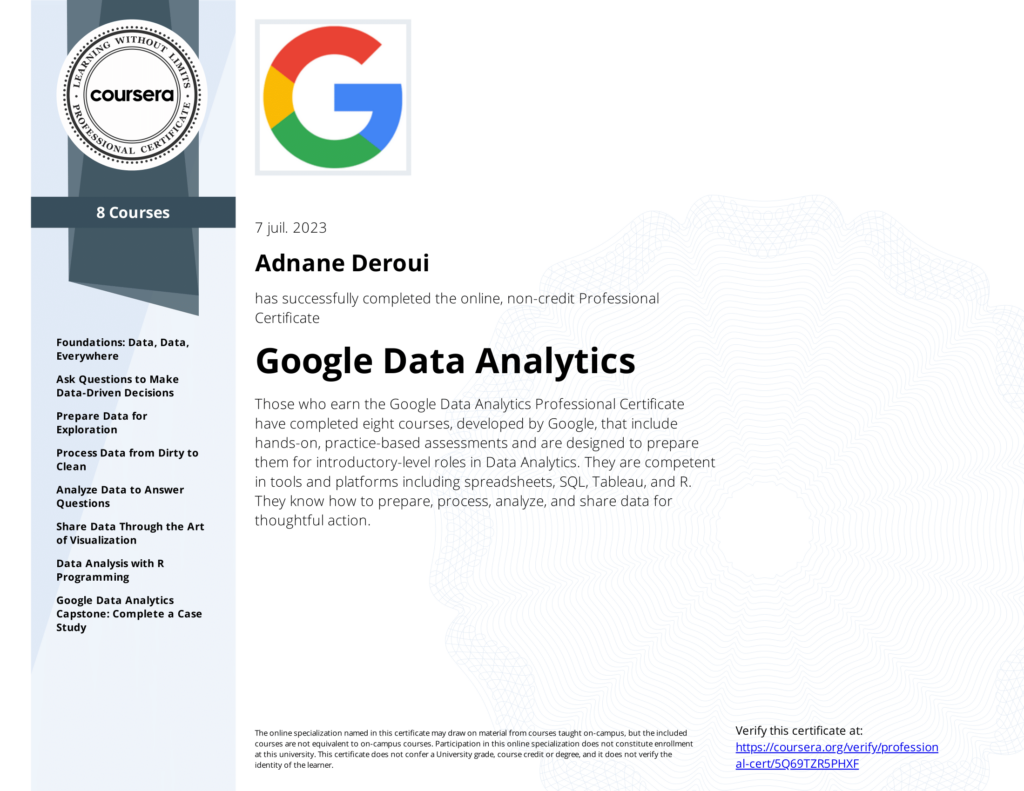
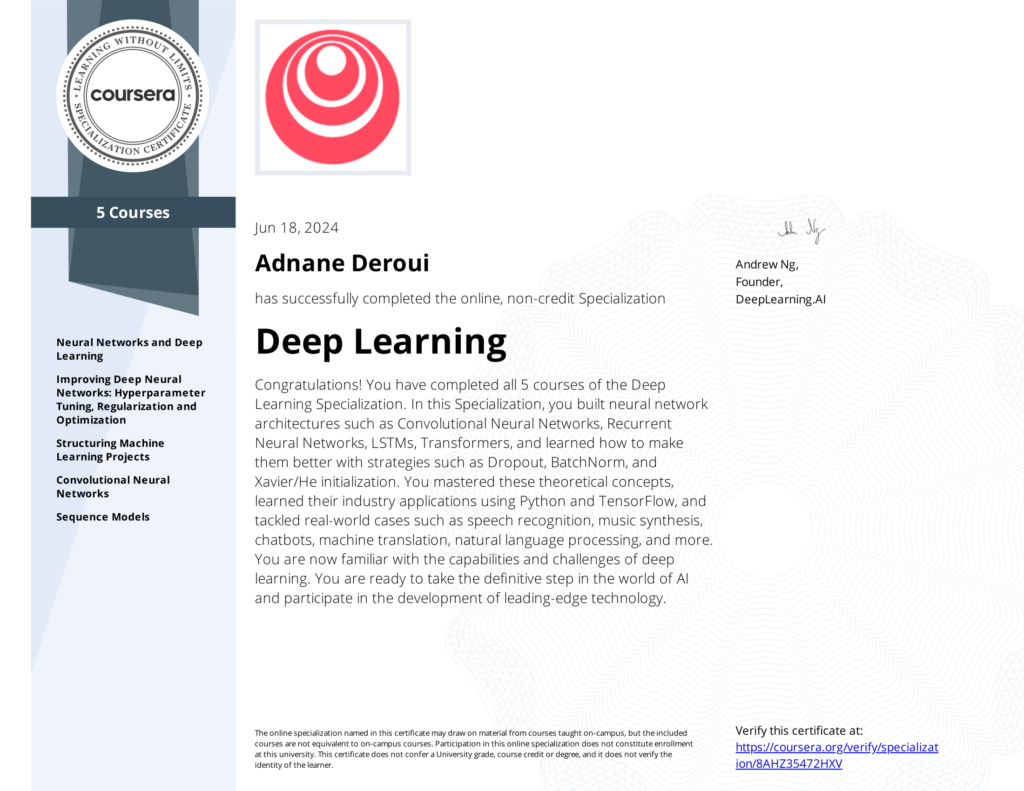


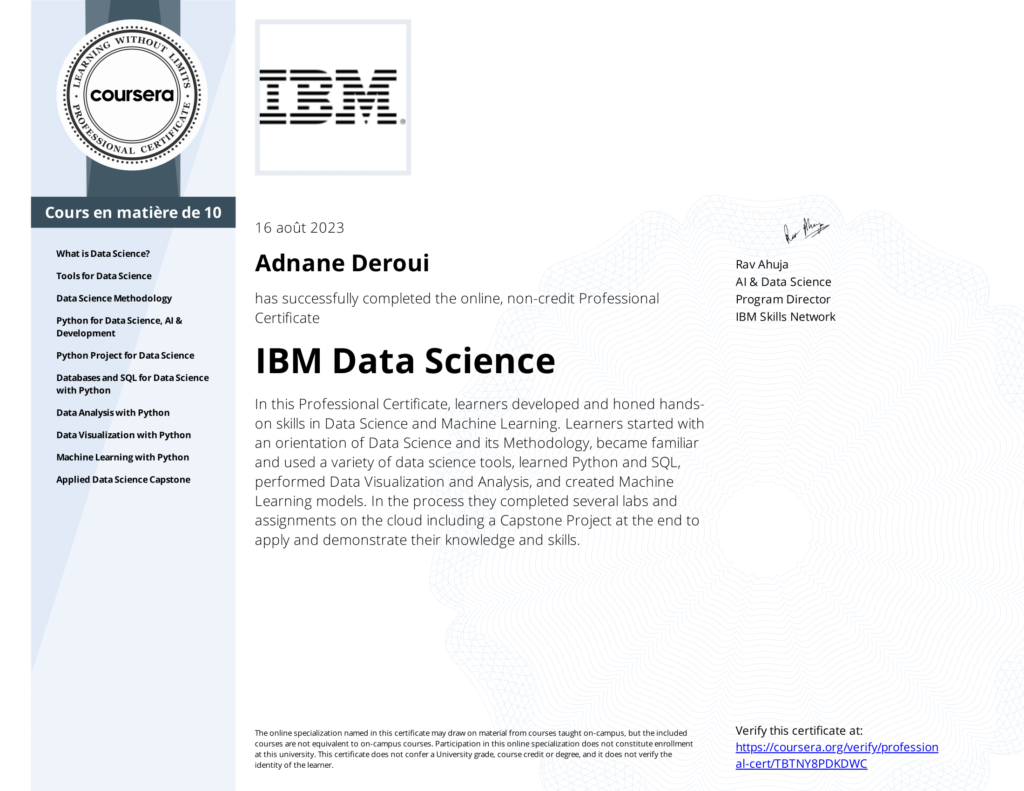
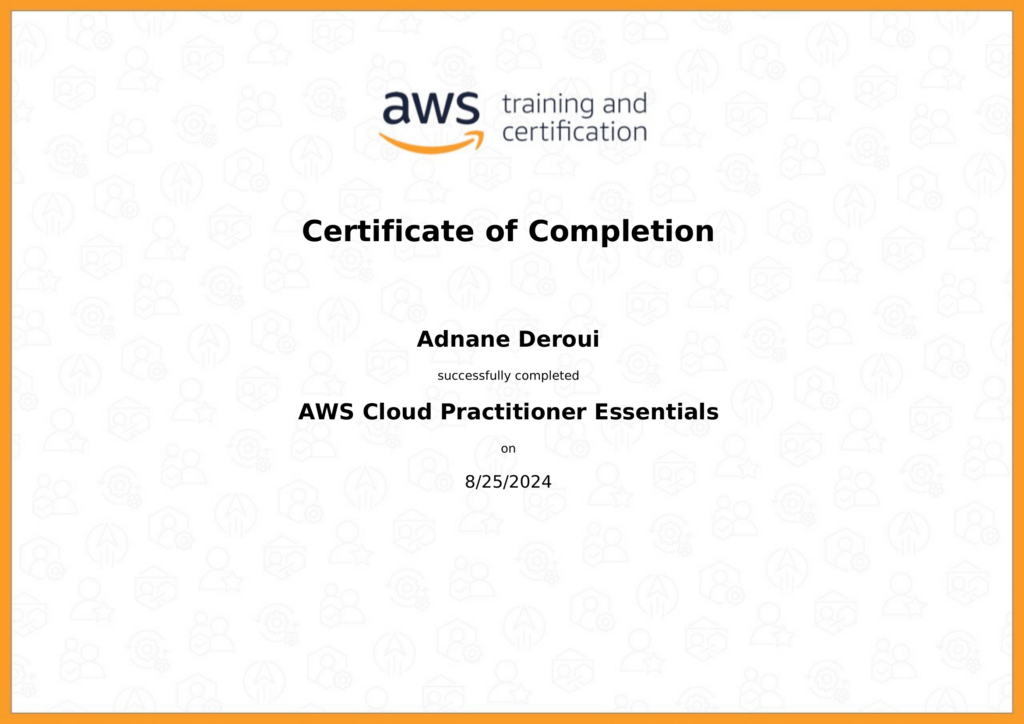
Work Experience
Data Science and Generative IA Intern:
- Performed exploratory data analysis using appropriate visualization techniques.
- Predict customer churn using the Random Forest algorithm, and optimize its hyperparameters.
- Extract data mainly from 10-K and 10-Q documents to develop a Large Language Model (LLM) capable of
making financial recommendations.
Final Year Project Intern:
- Participation in several regular controls of Wafacash agencies, including verification of security measures,
receipts, daily reports, as well as monitoring of KPIs. - Contribution to the development of a predictive model using statistical techniques, aimed at forecasting
the starting balance and monthly sales of each agency.
School Performance Tracking Intern:
- Collect, organize and regularly update student academic results and attendance rates using Microsoft
Excel. - Mobilize multiple Data Viz techniques to track student academic results, providing management with an
overview of class performance.
Academic Projects
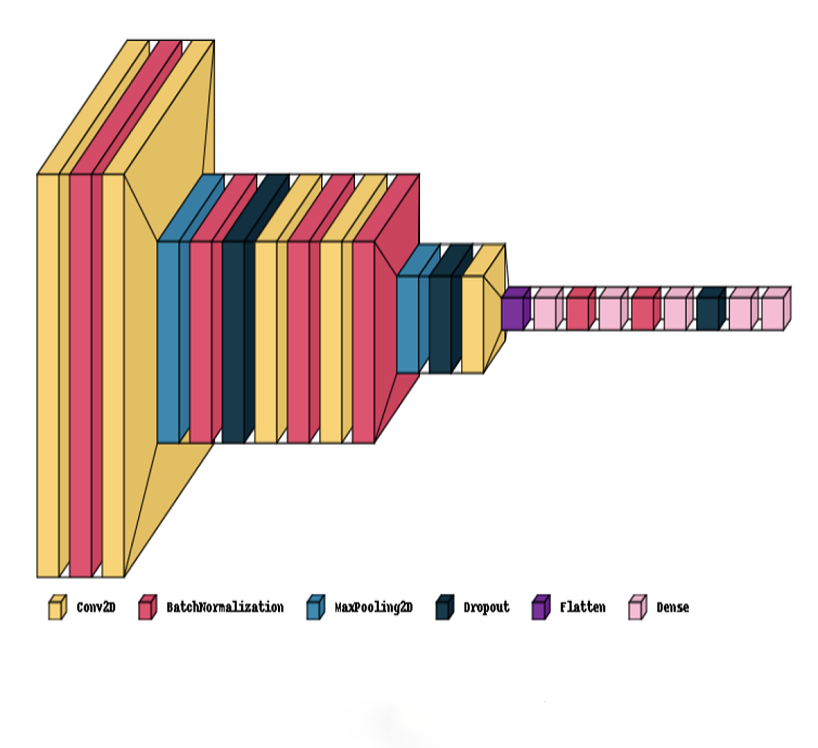
Detection of Invasive Ductal Carcinoma using a Deep Convolutional Network
This project focuses on automating the detection of Invasive Ductal Carcinoma (IDC), the most common form of breast cancer, using deep learning. We developed a convolutional neural network (CNN) to classify and localize cancerous tissue in histopathology images from over 277,000 patches. To address class imbalance and overfitting, we used data augmentation and model optimization techniques. Our CNN achieved 92% accuracy, demonstrating the potential of deep learning to streamline IDC diagnosis, improving both efficiency and precision in clinical settings.
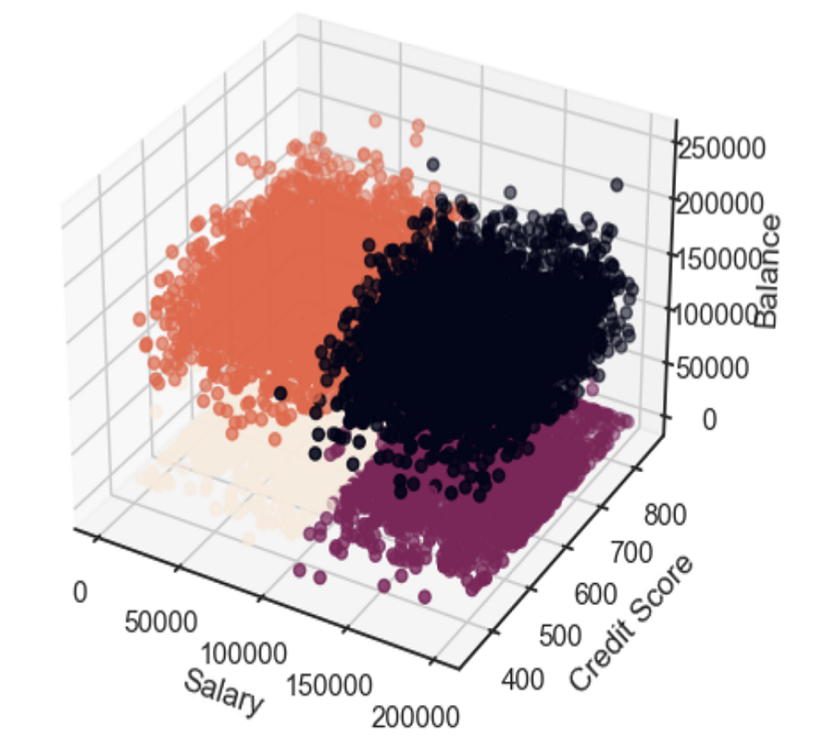
Banking Customer Segmentation using K-means Clustering
Customer Segmentation is a vital approach for banks to tailor their products and services to specific customer needs. Using K-means clustering, customers are grouped based on features such as age, balance, credit history, and loan status. This clustering method enables the identification of key segments, such as high-balance customers who are suitable for premium services, credit card offers, and personal loans, as well as customers with a history of defaults, who may benefit from debt management programs. By leveraging these clusters, banks can enhance customer targeting, delivering personalized banking products and services more effectively.
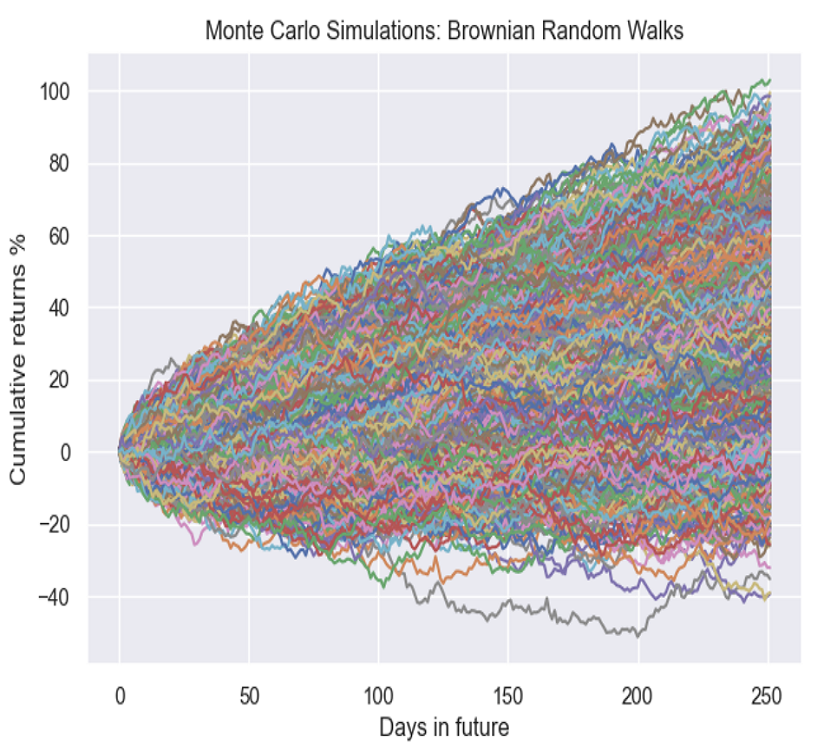
A Machine Learning Approach to Trend-Following Trading Strategies
In this project, we developed an algorithmic trading strategy utilizing a Deep Neural Network (DNN) to predict market trends and optimize portfolio returns. The strategy was rigorously backtested against historical market data, comparing it to a traditional buy-and-hold strategy. To assess performance, we analyzed key metrics such as cumulative returns, Sharpe ratios, and drawdowns. Additionally, Monte Carlo simulations were employed to generate 3,000 random paths based on Brownian motion, forecasting the next 252 trading days. The results highlighted the strategy’s robustness, with the simulated paths showing a median performance of 40% and moderate drawdowns, further supporting the strategy’s effectiveness in varying market conditions. The mean maximum drawdown across simulations was 12.5%, indicating controlled risk, while the DNN model achieved strong cumulative returns and favorable risk-adjusted performance.
VOLUNTEERING
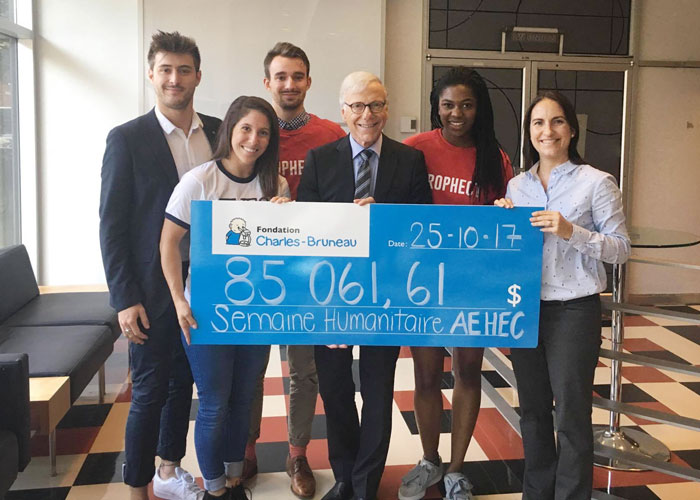
Équiterre, Montréal, Canada
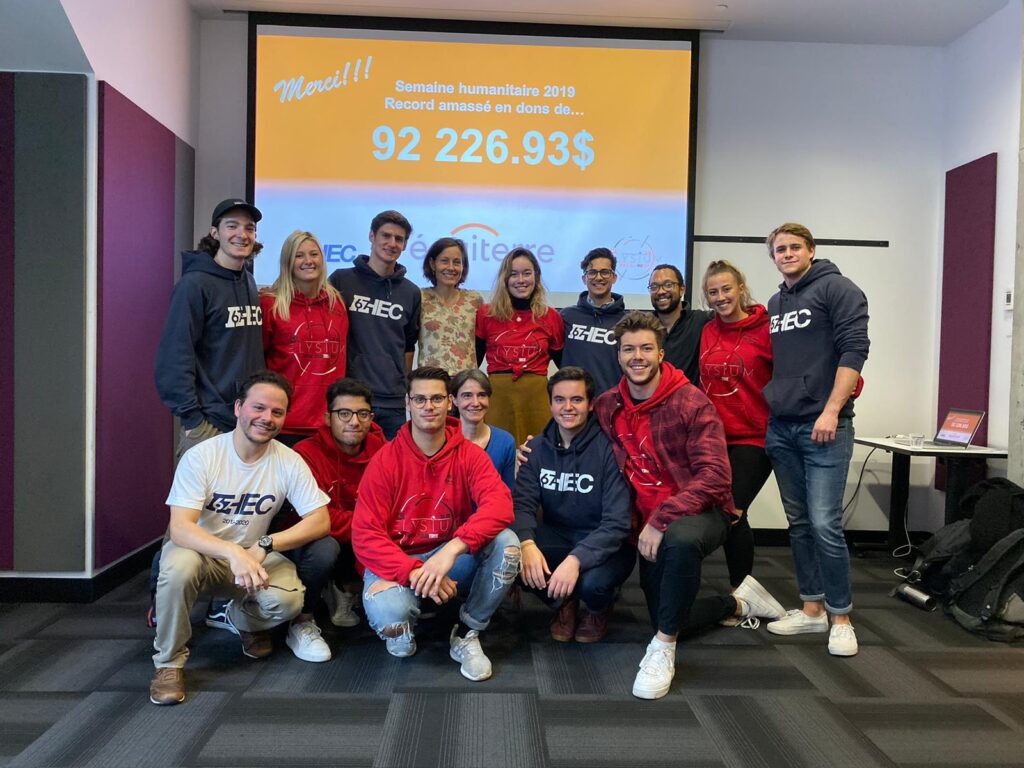